- The Fusionist
- Posts
- Predictive vs. Generative Design Methods for Design & Architecture
Predictive vs. Generative Design Methods for Design & Architecture
5 aspects for everyone to clarify expectations for digital tools that will shape the world of tomorrow, now.
Published in UX Collective, revised

image source: pexels.com
“For the first time, we have the power to decide the fate of our planet and ourselves. This is a time of great danger, but our species is young and curious and brave. It shows much promise.”
We have a crisis. There are less than 10 years left to meet 2030 goals, and according to the AIA 2030 report for 2019, only 15% of new projects reported are meeting those goals. There is a long way to go with limited resources. Fortunately, automation and computational design are catapulting designers, architects, and engineers into the exponential realm of digital modeling of complex systems for buildings, cities, and the environment. In the last 10 years alone, innovative technologies for performance-based design processes have advanced significantly in terms of multi-objective simulations, cloud computing, and machine learning. The result is that our current era proposes a new aesthetics and philosophy revolving around parametricism. Patrick Schumacher of Zaha Hadid Architects has fiercely pioneered this theory of parametric process as an aesthetic driver for avant-garde architecture. But what does this mean for the everyday designer, engineer, and architect?
Performance-based data-driven design tools push parametricism beyond aesthetics to evaluate the performance of a design in the near-real-time of its digital creation. These tools have many variations but can be categorized into two main groups that we’ll call predictive and generative design tools. As the AEC culture explores the various uses for each type, it is important to know how to examine them. Vetting a new digital process is possible by understanding what and how something is measured, what is assumed and why it matters, how to maintain quality and control, and ultimately expecting the appropriate return for the effort.
Knowing these key aspects of predictive and generative design processes will equip the everyday professional with a foundation, regardless of position or expertise, to understand what each tool can offer and which is better for them. Here are 5 key aspects of what to meaningfully expect from advanced computational modeling for predictive and generative design tools and how to frame the argument for predictive v. generative design results.
5 aspects of predictive and generative design methods to help clarify expectations.
Let’s set the stage.
I’m going to ignore the development of quantum computing here for simplicity and for the fact that there is only a handful in operation currently working, and none of them (that I know of) are analyzing buildings.
Gone are the days of simply drawing with the PC using software like AutoCAD. Here to stay are the complex building information models (BIM) in an era of computer-aided “everything.” It’s 2020, and I’ve just attended the latest conference for the Association for Computer-Aided Design in Architecture (ACADIA), a group of architects, designers, and artists all exploring the digital realm to find new ways to design and make things. It was particularly interesting this year as the whole event was a virtual conference presenting the latest projects and research that explore advanced computational processes for design. The irony did not escape me when we talked about advanced computational methods leveraging advanced computational infrastructure. The power of readily available digital hardware, international digital infrastructure, and cloud-based programs allowed video streaming discussions around the topic of other advanced digital tools.
Historically, faster computing power has offered promises of finally developing quantitative methods to measure qualitative outcomes, but with very few relevant developments, especially for the majority of architectural design. The closest example we have is computational heuristics, which is to say a computational method that aids discovery through trial and error of different parameters. However, just because that is how humans discover new insights doesn’t mean the computer is going to discover anything better using our method. Quicker, maybe (hopefully), but not necessarily better.
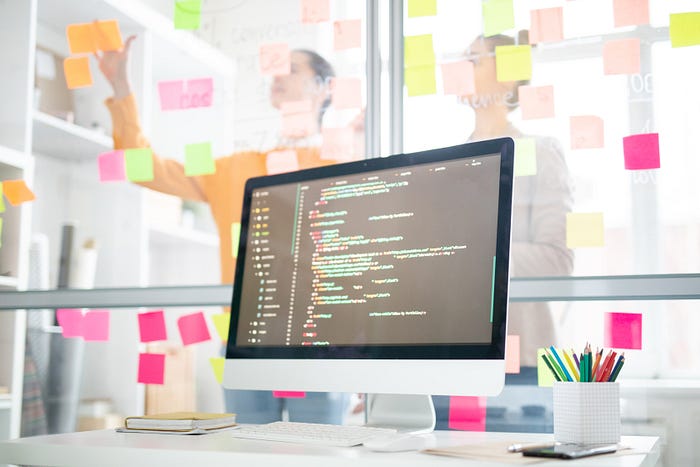
Let’s clarify a few things about our design methods before getting into the bits and pieces.
Data-driven design is an umbrella term describing a method of using collected information as input data to analyze and provide a more objective insight into what is happening that drives the design motive. Predictive and generative design are two examples of the spectrum of data-driven design methods using computational heuristics applied at various stages throughout the design processes. Each method has recently gained a lot of momentum (and money) for architectural design, so it’s worth taking a moment to clarify a few things.

Testing a current design to predict the social distancing potential of the layout, cove.tool.
Predictive design offers a critically different approach to achieving optimal design performance compared to generative design. On one end of the spectrum, predictive analysis tends to be in tandem with the designer throughout the process, allowing humans to determine the final shape or set of parameters. It essentially uses evidentiary information rather than speculative rationalization to determine the value of a solution. In other words, it tests a set of “functions” of a given “form” to see how it performs. The responsibility of determining a good or bad performance result is clearly on the user, not the computer, to determine if that design is the best fit to move forward.
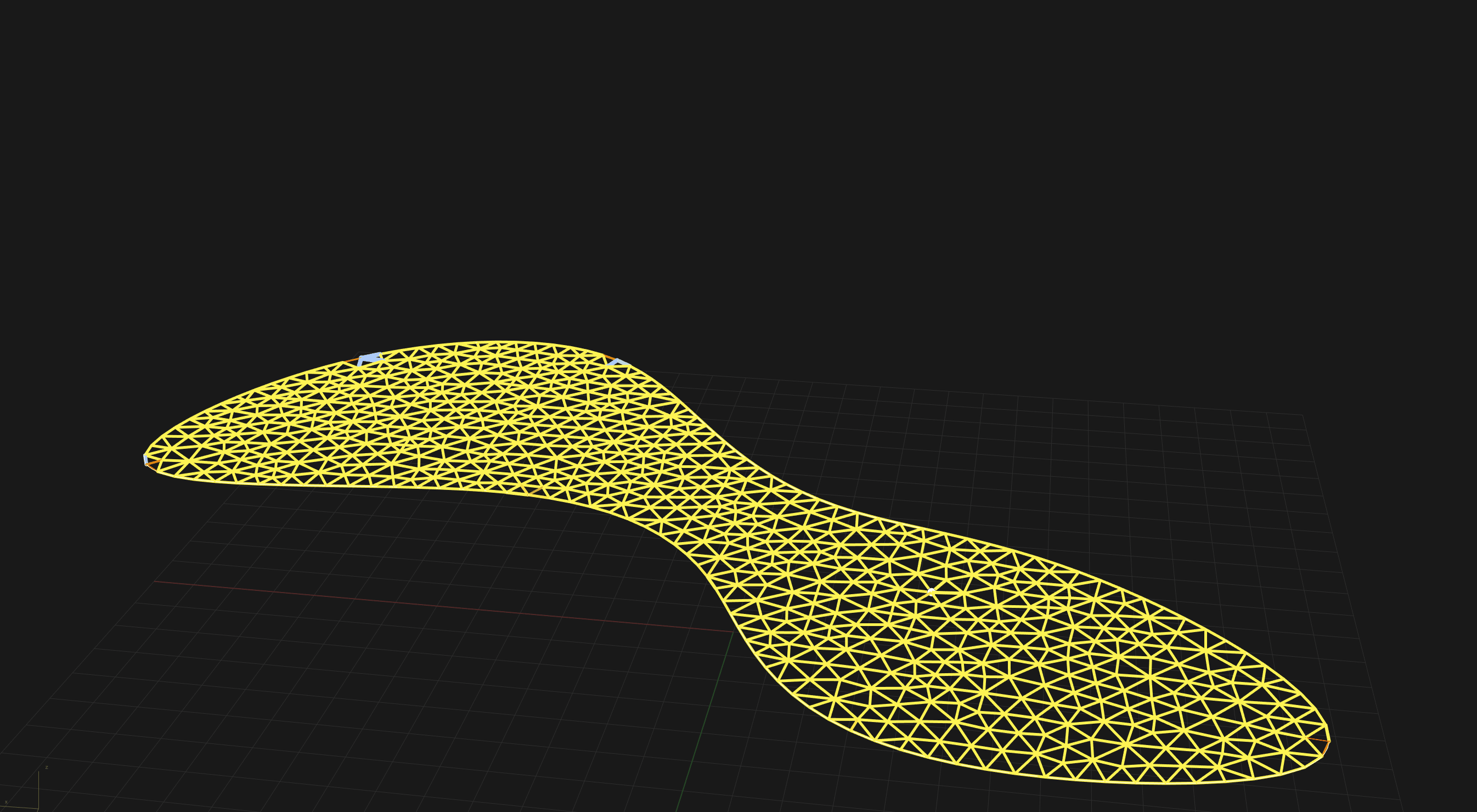
A Form-Finding method in Rhino3D using a Grasshopper script.
Reply